Why Business Will Be Negatively Affected by AI
Why business will be negatively affected by AI is a question demanding urgent attention. The rapid advancement of artificial intelligence presents both incredible opportunities and significant threats to businesses of all sizes. From job displacement caused by automation to the ethical dilemmas posed by biased algorithms, the impact of AI is multifaceted and potentially devastating if not carefully managed. This exploration delves into the key challenges businesses face in navigating this transformative technological landscape, examining the potential downsides and offering strategies for mitigation.
This analysis will dissect the various ways AI can negatively impact businesses, ranging from the immediate threat of job losses and increased competition to the long-term risks of ethical breaches and security vulnerabilities. We will explore the financial burdens of AI implementation, the challenges of integrating AI into existing infrastructure, and the critical need for human oversight to prevent unforeseen consequences. By understanding these potential pitfalls, businesses can proactively develop strategies to mitigate risks and harness the power of AI responsibly.
Job Displacement and Automation
The rise of artificial intelligence (AI) presents a significant challenge to the global workforce. AI’s capacity to automate tasks previously requiring human intervention is rapidly transforming industries, leading to concerns about widespread job displacement and its associated economic consequences. This section will explore the potential impact of AI-driven automation on various sectors, focusing on the vulnerability of different skill levels and proposing mitigation strategies to address the challenges faced by displaced workers.
AI’s Impact Across Sectors
AI’s automation potential varies significantly across different sectors. While some industries, like manufacturing and transportation, have already experienced substantial automation, other sectors, such as healthcare and education, are beginning to see the integration of AI technologies, potentially leading to future job displacement. The economic consequences of widespread job losses could include increased unemployment, widening income inequality, and social unrest. Specific roles at risk include data entry clerks, telemarketers, truck drivers, and even some roles in customer service and accounting. These roles often involve repetitive, rule-based tasks easily automated by AI systems. The resulting economic fallout could be substantial, requiring proactive measures to mitigate its impact.
Vulnerability of Different Skill Levels
AI-driven automation disproportionately affects certain skill levels. Low-skill jobs, often involving routine and manual tasks, are the most vulnerable to automation. Conversely, high-skill jobs requiring complex problem-solving, critical thinking, and creativity are less susceptible to immediate replacement. However, even high-skill jobs may see some tasks automated, necessitating adaptation and upskilling. Mid-skill jobs, those requiring specialized knowledge and skills but not necessarily high levels of critical thinking, are also at risk. The transition will require significant investment in education and retraining to equip workers with the skills needed for the evolving job market.
Job Category | Automation Potential | Impact on Employment | Mitigation Strategies |
---|---|---|---|
Data Entry Clerk | High | Significant job losses | Retraining in data analysis, programming, or project management |
Truck Driver | High (long-haul trucking) | Significant job losses in specific segments | Retraining in logistics management, drone operation, or maintenance of autonomous vehicles |
Customer Service Representative | Medium (some tasks automatable) | Shift towards higher-skill roles requiring empathy and complex problem-solving | Upskilling in conflict resolution, emotional intelligence, and advanced customer relationship management |
Software Developer | Low | Potential for increased demand due to AI development | Continuous learning and specialization in AI-related fields |
Physician (diagnostic tasks) | Medium (certain diagnostic tasks automatable) | Increased efficiency and potential for more patient interaction | Focus on patient care, complex diagnoses requiring human judgment, and ethical considerations of AI in healthcare |
Challenges and Retraining Programs
Displaced workers face numerous challenges in adapting to a changing job market. These challenges include a lack of transferable skills, age discrimination, and geographical limitations. Retraining programs are crucial to address these challenges. Effective programs should be tailored to individual needs, offering flexible learning pathways, and providing financial support to participants. These programs should focus on in-demand skills, such as programming, data analysis, cybersecurity, and digital marketing. Furthermore, partnerships between educational institutions, businesses, and government agencies are vital to ensure the effectiveness and accessibility of retraining initiatives. Successful programs should also emphasize soft skills like communication, teamwork, and problem-solving, which are increasingly important in the AI-driven economy. Examples of successful retraining programs include those focused on reskilling manufacturing workers for roles in advanced manufacturing and those supporting the transition of transportation workers into logistics management. These programs often incorporate apprenticeships, online courses, and on-the-job training to maximize their impact.
Increased Competition and Market Disruption

The rise of AI is fundamentally reshaping competitive landscapes across numerous industries. Businesses leveraging AI technologies are gaining significant advantages, leading to increased market disruption and posing considerable challenges for those unable to adapt. This competitive shift stems primarily from enhanced efficiency, reduced operational costs, and the creation of entirely new market opportunities.
AI-powered businesses often demonstrate significantly higher efficiency and lower operational costs compared to their traditional counterparts. This advantage is driven by AI’s ability to automate tasks, optimize processes, and analyze vast datasets to make data-driven decisions. Automation reduces labor costs, minimizes errors, and speeds up production cycles, leading to a substantial competitive edge. For example, AI-powered chatbots can handle customer service inquiries 24/7, reducing the need for large customer service teams, while AI-driven predictive maintenance can prevent costly equipment downtime in manufacturing. This translates directly into higher profit margins and the ability to offer more competitive pricing.
Industries Significantly Impacted by AI-Driven Competition
The impact of AI on competition is already evident in several sectors, with further disruption predicted across a wider range.
- Retail: AI-powered recommendation systems personalize shopping experiences, leading to increased sales and customer loyalty for companies like Amazon. Traditional retailers struggle to compete with this level of personalized marketing.
- Finance: AI algorithms are used for fraud detection, risk assessment, and algorithmic trading, giving financial institutions using AI a significant edge in efficiency and profitability. Smaller firms without access to these technologies face a disadvantage.
- Healthcare: AI-powered diagnostic tools and personalized medicine are transforming healthcare, enabling faster and more accurate diagnoses. Hospitals and clinics that fail to adopt these technologies may fall behind their competitors.
- Transportation: The development of self-driving vehicles is poised to disrupt the transportation industry, impacting trucking, ride-sharing, and logistics companies. Companies slow to adopt autonomous technology risk obsolescence.
Challenges Faced by SMEs in Competing with AI-Enabled Corporations
Small and medium-sized enterprises (SMEs) often face significant hurdles in competing with larger corporations that have the resources to invest in and implement AI technologies. These challenges include:
- High initial investment costs: Developing and implementing AI solutions requires substantial upfront investment in software, hardware, and skilled personnel, which can be prohibitive for SMEs with limited budgets.
- Lack of skilled workforce: Finding and retaining employees with the necessary AI expertise can be difficult for SMEs, especially in competitive job markets.
- Data scarcity: AI algorithms require large amounts of data to function effectively. SMEs often lack the data volume necessary to train and optimize their AI systems to the same level as larger corporations.
- Integration challenges: Integrating AI solutions into existing business processes can be complex and time-consuming, requiring significant technical expertise and resources.
Ethical Concerns and Bias in AI Systems: Why Business Will Be Negatively Affected By Ai
The increasing integration of artificial intelligence (AI) into business operations presents significant ethical challenges. These concerns stem from the inherent biases embedded within AI systems and the potential for these systems to exacerbate existing societal inequalities, raising serious questions about fairness, accountability, and transparency. Ignoring these ethical implications can lead to reputational damage, legal repercussions, and a loss of customer trust.
Algorithmic bias and data privacy are paramount ethical considerations. AI algorithms are trained on data, and if that data reflects existing societal biases, the algorithm will likely perpetuate and even amplify those biases. This can lead to discriminatory outcomes in areas such as hiring, loan applications, and even criminal justice. Simultaneously, the collection and use of personal data to train and operate AI systems raise significant privacy concerns, particularly regarding the potential for misuse or unauthorized access.
Algorithmic Bias and its Societal Impact
AI systems, being products of their training data, can inherit and amplify pre-existing biases present in society. For example, if a facial recognition system is trained primarily on images of individuals from a particular demographic, it may perform poorly when identifying individuals from other demographics, leading to misidentification and potentially discriminatory outcomes. Similarly, an AI-powered hiring tool trained on historical hiring data that reflects gender or racial bias may perpetuate those biases by unfairly favoring certain candidates over others. This can lead to significant disadvantages for underrepresented groups and deepen existing societal inequalities. The lack of diversity in the datasets used to train AI systems further exacerbates this issue.
Data Privacy and Security in AI Systems
The use of AI in business often involves the collection and analysis of large amounts of personal data. This raises significant concerns about data privacy and security. For instance, AI-powered customer service chatbots may collect sensitive information about customers’ preferences, financial situations, and even health conditions. If this data is not properly secured, it could be vulnerable to breaches, leading to identity theft, financial loss, and reputational damage for the business. Furthermore, the use of AI for surveillance purposes raises concerns about the potential for mass surveillance and erosion of individual liberties.
Mitigating Ethical Risks in AI Implementation
Businesses can take several steps to mitigate the ethical risks associated with AI. This involves a multi-faceted approach encompassing data governance, algorithm transparency, and ongoing monitoring and evaluation.
Ethical AI Implementation Decision-Making Process
A robust decision-making process is crucial for the ethical implementation of AI. The following flowchart Artikels a potential approach:
[Flowchart Description: The flowchart would visually represent a decision tree. It would start with a central question: “Is the AI application necessary and beneficial?” A “yes” answer would lead to a series of checks: “Is the data used representative and unbiased?” “Are privacy concerns addressed?” “Are appropriate safeguards in place to prevent bias and discrimination?” “Is there a mechanism for accountability and redress?” A “no” answer to any of these would lead to a “re-evaluate application” branch. A “yes” answer to all would lead to an “Implement AI application” branch. A “no” answer to the initial question would lead to a “Do not proceed” branch.]
This structured approach helps ensure that ethical considerations are prioritized throughout the AI lifecycle, from initial conception to ongoing monitoring. Regular audits, independent evaluations, and continuous improvement are essential to maintain ethical standards and address emerging challenges.
High Initial Investment Costs and Implementation Challenges
The adoption of artificial intelligence (AI) presents significant hurdles for businesses, extending beyond the readily apparent concerns of job displacement and ethical dilemmas. A major obstacle lies in the substantial upfront investment and the complex integration process required to successfully implement AI technologies. This necessitates a careful evaluation of potential returns and a realistic assessment of the challenges involved.
The financial commitment needed for AI integration is substantial, encompassing not only the cost of advanced software and powerful hardware but also the salaries of highly skilled data scientists, engineers, and AI specialists needed to develop, deploy, and maintain these systems. This investment can be prohibitive for smaller businesses and even present a considerable strain on larger corporations, particularly when considering the potential for unsuccessful implementations. Furthermore, ongoing maintenance and updates add to the long-term financial burden.
AI Implementation Costs and Resource Requirements
Implementing AI involves significant expenditure across several key areas. Software licenses for machine learning platforms, deep learning frameworks, and specialized AI tools can be extremely costly. High-performance computing hardware, including powerful servers, GPUs, and specialized AI chips, is essential for processing large datasets and running complex algorithms. This hardware often requires significant upfront capital investment and ongoing maintenance costs. Beyond these tangible expenses, the recruitment and retention of skilled AI professionals represent a significant and ongoing financial burden. Competition for talent in this field is fierce, driving up salaries and making it difficult for businesses to build and maintain competent AI teams.
Technical Challenges of AI Integration
Integrating AI systems into existing business processes and infrastructure presents considerable technical challenges. Legacy systems may not be compatible with modern AI technologies, requiring significant upgrades or replacements. Data integration can be a major bottleneck, as AI systems require large, clean, and well-structured datasets for effective training and operation. Data cleansing, transformation, and integration processes can be time-consuming and resource-intensive. Furthermore, ensuring data security and privacy is crucial, requiring robust security measures to protect sensitive information. The complexity of AI algorithms and the need for continuous monitoring and fine-tuning can also lead to significant technical challenges. Successfully navigating these complexities requires a deep understanding of both AI technologies and the specific business context.
Return on Investment (ROI) of AI Across Industries
The ROI of AI implementation varies significantly across different industries and depends heavily on factors such as the specific application, data quality, and the business’s ability to effectively integrate and utilize the technology. While some industries see rapid and substantial returns, others may experience slower adoption or even unsuccessful implementations.
Industry | ROI | Success Factors | Challenges |
---|---|---|---|
Financial Services | High (potential for significant automation and improved efficiency) | Clear business objectives, high-quality data, skilled workforce | Regulatory compliance, data security concerns, high initial investment |
Healthcare | Moderate (potential for improved diagnostics and personalized medicine) | Strong collaboration between clinicians and data scientists, ethical considerations addressed | Data privacy regulations, integration with existing systems, high implementation costs |
Manufacturing | Moderate to High (potential for increased productivity and reduced costs) | Integration with existing automation systems, skilled workforce, clear ROI metrics | Data acquisition and quality, legacy system compatibility, high upfront investment |
Retail | Moderate (potential for improved customer experience and targeted marketing) | Customer data integration, effective personalization strategies, clear business objectives | Data privacy concerns, competition from established players, high initial investment |
Security Risks and Data Breaches

The increasing reliance on AI systems by businesses introduces significant security vulnerabilities. AI systems often handle vast quantities of sensitive data, making them attractive targets for cyberattacks. A successful breach can not only lead to direct financial losses but also severely damage a company’s reputation and expose it to substantial legal liabilities. The complexity of AI systems, coupled with the potential for unforeseen vulnerabilities in their algorithms and underlying infrastructure, further exacerbates these risks.
The potential consequences of a data breach stemming from compromised AI systems are far-reaching and devastating. Financial losses can be substantial, encompassing costs associated with incident response, legal fees, regulatory fines, and the loss of revenue due to business disruption. Reputational damage can be equally, if not more, crippling, leading to loss of customer trust, decreased brand loyalty, and difficulty attracting new business. Furthermore, businesses face significant legal liabilities, including potential lawsuits from affected customers and regulatory penalties for non-compliance with data protection laws like GDPR or CCPA. The scale of these consequences underscores the critical need for robust security measures.
Strategies for Protecting AI Systems and Data, Why business will be negatively affected by ai
Effective protection of AI systems and data requires a multi-layered approach that integrates various security strategies. These strategies must be proactively implemented and continuously monitored to adapt to the ever-evolving threat landscape.
- Robust Data Encryption: Implementing strong encryption protocols for both data at rest and data in transit is paramount. This ensures that even if a breach occurs, the data remains inaccessible to unauthorized individuals. This includes employing encryption algorithms that are resistant to known attacks and regularly updating encryption keys.
- Regular Security Audits and Penetration Testing: Regular security assessments, including penetration testing, are crucial for identifying vulnerabilities in AI systems and their supporting infrastructure. These assessments should be conducted by independent security experts to provide an unbiased evaluation of the system’s security posture. Findings should be addressed promptly and effectively.
- Intrusion Detection and Prevention Systems (IDPS): Deploying sophisticated IDPS solutions is essential for monitoring network traffic and identifying malicious activities in real-time. These systems should be configured to detect and respond to various types of attacks, including those specifically targeting AI systems, such as adversarial attacks or data poisoning.
- Access Control and Authentication: Implementing strict access control measures is vital to limit access to sensitive data and AI systems to authorized personnel only. Multi-factor authentication (MFA) should be mandatory for all users, adding an extra layer of security to prevent unauthorized access.
- Employee Training and Awareness: Educating employees about cybersecurity best practices and the importance of data protection is crucial. Regular training programs should be conducted to raise awareness of phishing scams, social engineering attacks, and other common threats. This proactive approach helps prevent human error, a major cause of many security breaches.
Dependence and Lack of Human Oversight

Over-reliance on AI systems, while promising efficiency gains, introduces significant risks, especially in scenarios demanding nuanced human judgment and critical thinking. The inherent limitations of current AI technologies, coupled with the potential for unforeseen biases and errors, necessitate a careful balance between automation and human oversight to mitigate negative consequences for businesses. Failing to maintain this balance can lead to costly mistakes, reputational damage, and ultimately, business failure.
The core issue lies in the potential for AI systems to become “black boxes,” where the decision-making process is opaque and difficult for humans to understand or challenge. This lack of transparency can mask errors and biases, leading to unfair or inaccurate outcomes. Furthermore, the tendency to trust AI outputs implicitly, without critical evaluation, can lead to the acceptance of flawed decisions with potentially severe repercussions. Maintaining human oversight is crucial not only to detect and correct these errors but also to ensure that AI systems align with ethical standards and business objectives.
Consequences of Insufficient Human Oversight in AI Systems
Insufficient human oversight in AI-driven processes can result in a range of negative consequences for businesses. These consequences extend beyond simple operational inefficiencies and encompass ethical breaches, legal liabilities, and significant financial losses. For instance, relying solely on AI for customer service without human intervention might lead to frustrated customers due to the inability of the AI to handle complex or nuanced requests. In financial institutions, algorithmic trading systems operating without adequate human supervision could trigger market instability or lead to substantial financial losses due to unforeseen market fluctuations or algorithmic errors. Similarly, in healthcare, AI-driven diagnostic tools without human review could lead to misdiagnosis and potentially life-threatening consequences.
Examples of Negative Impacts from Over-Dependence on AI
Consider a scenario where a retail company relies entirely on an AI-powered system to manage its inventory. If the AI system malfunctions due to a software glitch or inaccurate data input, it could lead to stockouts of popular products or overstocking of less-demanding items, resulting in lost sales and increased storage costs. Another example is a recruitment firm using AI to screen job applications. Without human review, the AI might inadvertently discriminate against certain demographic groups due to biases present in the training data, leading to legal challenges and reputational damage. In the autonomous vehicle sector, a failure in the AI system’s decision-making process, without human intervention, could result in accidents with significant safety and liability implications. These examples highlight the critical need for human oversight to ensure the responsible and effective implementation of AI systems across various business functions.
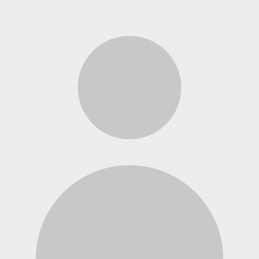
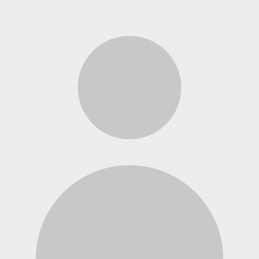
What's Your Reaction?
-
Like
-
Dislike
-
Funny
-
Angry
-
Sad
-
Wow